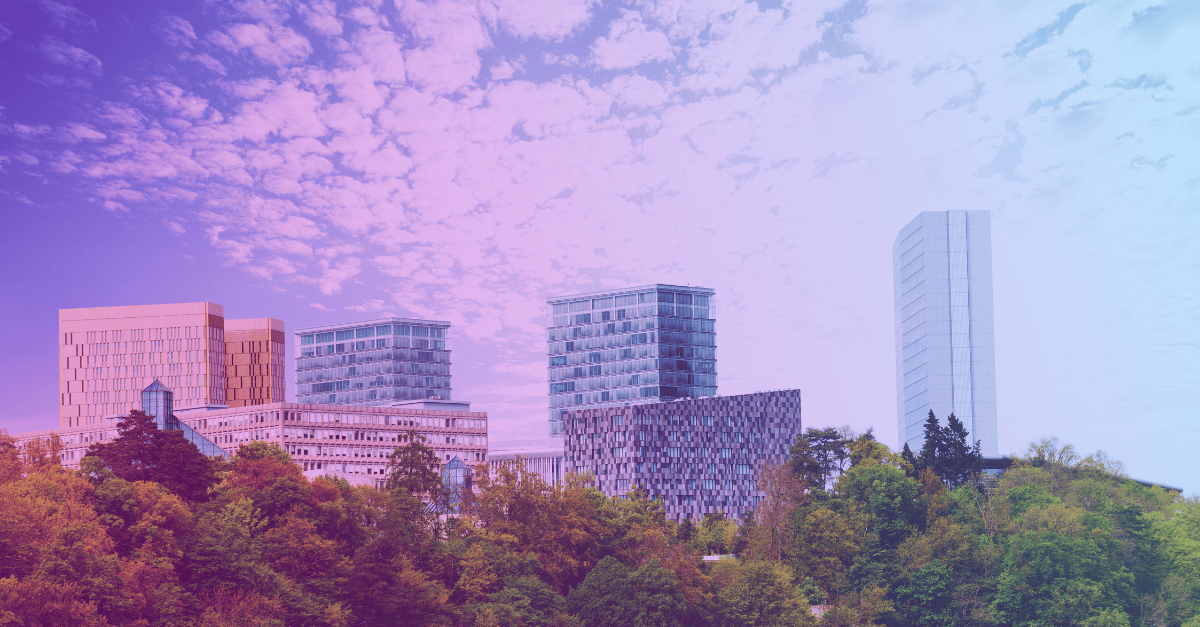
Revolutionizing Financial Data Processing with MeluXina: Transforming Luxembourg’s Financial Ecosystem
Luxembourg is recognized as a global financial hub, renowned for its innovation, stability, and regulatory environment. In recent years, the integration of high-performance computing (HPC) has become a crucial component of maintaining and enhancing this status. Central to this technological advancement is MeluXina, Luxembourg’s state-of-the-art supercomputer, operated by LuxProvide. MeluXina is not just a technical achievement; it is a catalyst for profound change in the financial sector, enabling institutions to process and analyze vast datasets with unprecedented speed and accuracy.
To understand how MeluXina and LuxProvide can drive this change, we will look at what can MeluXina and LuxProvide do (in general), and what can MeluXina and LuxProvide do for finance?
What can MeluXina and LuxProvide do?
Let us start with the (shortest possible) overview of HPC. A long time ago, HPC meant a specific, sophisticated, machine and software (the supercomputer). Mass market dynamics, the Internet revolution and cooling issues have now redefined HPC to become a large collection of PCs (personal computers), running PC operating systems, talking common Internet language. Of course, these PCs are not quite consumer grade PC, come with high-end accelerators, connected with optic fibers, stacked in racks, liquid cooled, animated with colorful, flashing LEDs.
However short and entertaining, this overview of HPC remains technical; it is too far from financial applications. Before enumerating these applications, we can abstract HPC and MeluXina to their capability: what kind of computation is possible? We propose to classify HPC computations into the 3 types below:
1. Compute as an Oracle (we compute for an answer), this is the traditional use-case for HPC. Input is a large data set, a program or model. Output is data, typically much smaller than the input:
- A large computation, whose results hold insights into a problem or model.
- The stress test of a compute-intensive software or model. Output is performance or accuracy measure.
- A pattern detection program on a large data set. Output is the patterns detected.
- The processing of large data sets, such as social media feeds.
2. Compute as a Service, a variant of the Oracle. The result is still an answer, but the execution is more frequent, and the response is expected sooner. This capability is closer to the Cloud. The input is a smaller data set and output is the same as for the Oracle:
- The offload, on-demand, of an intensive computation that does not fit well with the Cloud resources.
- A common example is AI inference (which may require powerful GPUs, available on MeluXina).
3. Compute as a Factory, input is still a large data set and a program, but the output is another program. The program-as-output is then used to provide answers to future questions. The Factory kind of computing, despite consuming many resources, is still cost-effective, because resources are only needed at design time. The output program is expected to be sufficiently lightweight to be deployed on a smaller infrastructure.
- A widespread application is training an AI model, using statistical machine learning methods (output is a trained model).
- Factory outputs are sometimes called policies or strategies, the key part of an otherwise standard program that captures the essential function.
- But why stop here? Output can be actual program code, such as source code inferred by Large Language Model, a heuristic (fast and frugal program dedicated to a problem) discovered by a search algorithm or another version of the input program with a different profile (mobile version or an FPGA version).
MeluXina can be your design tool.
Hopefully this clarifies what HPC can do for your business. Next, we list several applications for finance, mapping each to the 3 types of computation above.
What can MeluXina and LuxProvide do for finance?
In a similar pattern to other fields (engineering, physics, chemistry), computational finance represents the shift from pure mathematical methods (manipulating closed forms) to computer-based numerical methods. This approach is now widespread in finance and has expanded to require significant computing resources: MeluXina is the ideal platform for this task, because it provides high-performance computing (including GPU and FPGA) on demand.
Let’s look at examples of computational finance applications and how they relate to the 3 kinds of HPC computation introduced previously: Oracle, Service and Factory.
Options pricing
Options pricing are estimated using numerical methods, such as Lattice models (binomial, trinomial) and Monte-Carlo simulations. The computation involves establishing some probability measures and then numerically simulating with the resulting stochastic processes. HPC can address the growing computation load of these methods, due to increasing quantity of data per asset (observations), option complexity and number of assets. MeluXina offers many-core nodes, several accelerators, fast interconnects and software libraries well suited for this (see Nvidia GPU Gems examples).
Options pricing maps to all 3 kinds of HPC computations: executing a pricing algorithm fits the Oracle type because the output is a price (data). A need for faster response times, as a service, can point to the Service type. The recent development of Deep Learning and other machine learning approaches point to the third type of HPC computation, Factory, when a model for pricing is to be trained (or fine-tuned) first.
Algorithmic trading strategies
Here, algorithmic trading refers to automated trade decision-making, but not the high-frequency trading which is considered as the execution of a decided trade (using many small transactions).
A given algorithmic strategy can be simulated (tested) at scale on an HPC, mapping to an Oracle type of computation. More complex strategies, based on large data sets, or more creative strategies, can obviously be designed by computation, mapping this application to the Factory kind. It is the clearest example of a Factory type of HPC computation because many AI approaches aim to define a policy. Most of the artificially designed methods, such as pattern-matching, Markov Chain Monte Carlo, Reinforcement Learning and other AI approaches, are usually compute intensive and therefore well-suited to MeluXina.
The operation of an already designed algorithmic trading system, while potentially processing large amounts of data, could benefit from an HPC environment, however the desired response time and immediate availability are not a good match to HPC.
Portfolio management
Portfolio management is the design, over time, of asset allocation. The objectives are to maximize the expected return while minimizing risk. This problem relies on individual asset pricing (such as the derivatives above), but introduces a specific challenge, a multi-objective optimization* problem (we’re looking for a good portfolio after all).
Techniques include linear/quadratic/non-linear programming, metaheuristics, stochastic methods and statistical machine learning (for example: deep learning). All of which are computation intensive tasks and therefore well suited to MeluXina.
From the previous examples, we expect portfolio management to use all 3 types of HPC computation: Oracle and Service when a data result is needed (for example: scoring a portfolio, selection of asset classes, alarm prompting portfolio update), Factory when a portfolio management tool is the target. This tool is then run on a more common computer to obtain answers cost-effectively.
Risk management
The recent financial crises and the increased regulation that followed, have made managing credit, operational and market risk more computation intensive and complex. The risk is inherent to trading and portfolio management (position limits and sector exposures, liquidity ratios, private equity recommitment), but also covers bank stress tests, anti-money laundering and fraud detection.
Some of these activities involve significant computation and large data sets, all 3 types of HPC computation are possible: the Oracle type when a large computation is run occasionally and results in a single answer (for example: a stress test result), or the Service type when more frequent and faster results are expected. Finally, the Factory kind of computation is used when training an AI model to detect fraudulent transactions or when discovering a risk formula.
Take-away
Computational finance numerical methods, the digitalization trend and the increasingly complex environment all conspire to demand more computation. Also, increased computing power inspires more demanding algorithms. We could therefore feel pessimistic about meeting our objectives.
HPC, and MeluXina in particular, can nevertheless give you a computing edge, essentially because of those colorful, flashing LEDs, liquid cooling, racks… because of the focus on performance.
Focusing on performance often sacrifices ease-of-use, but as we have seen, HPC components are similar to commodity technical parts, to which we are accustomed (besides, LuxProvide also provides HPC consulting).
Financial innovation
To conclude, we must highlight that all the above financial activities are never permanently solved, in part due to the evolving regulatory environment, but more so because of the constant innovation in financial markets. Financial innovations need to be designed, priced and capitalized, and finally traded in or out of portfolios. Innovations are also increasingly complex, all of which translates to increased computational needs.
Luxembourg’s financial center (international fund management, capital market, regulation framework) is always subject to global competition, yet the increasing computing power and advances in AI (and not limited to the deep learning flavored AI), points to artificially designed financial innovation; the Factory kind of HPC:
“Financial economists are called upon not only to value the new instruments and the new dynamic trading strategies that make heavy use of the new instruments but to design them as well. Like engineers who use physics, financial engineers use the techniques of modern finance to build the equivalent of bridges and airplanes.” [1] (to be fair, the quote does not mention other key factors to innovation: it is also an art and an economic activity)
(*) Optimization means good enough, because of bounded rationality.
[1] Stavros A. Zenios, “High-performance computing in finance: The last 10 years and the next”, Parallel Computing, Volume 25, Issues 13–14, pp. 2149-2175, 1999.
About the author
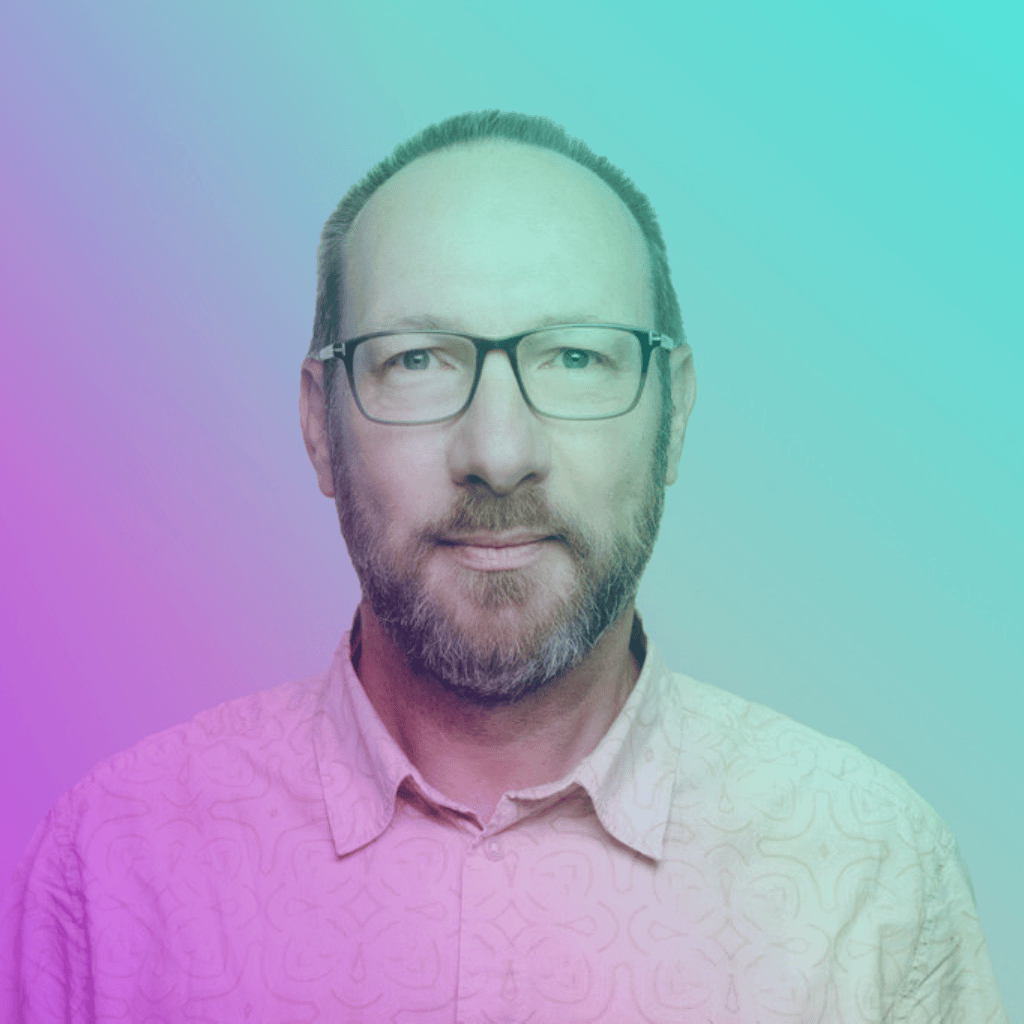
Ready to push the boundaries of digital innovation?
Reach out to us now and let’s make it happen.
ul.px1734838439l@ofn1734838439i1734838439
(+352) 85 99 14
OFFICES
ATRIUM BUSINESS PARK
31, Rue du Puits Romain
L-8070 Bertrange
Luxembourg
MELUXINA
SUPERCOMPUTER
LUXCONNECT DC2
3 Op der Poukewiss
7795 Bissen
Luxembourg